- Home
- POINT OF VIEWS
The Winning Integration: How Predictive Maintenance Supports Annex 1 in Pharmaceutical Manufacturing
Paolo Alzalamira
R&D and Technical Manager at Dos&Donts
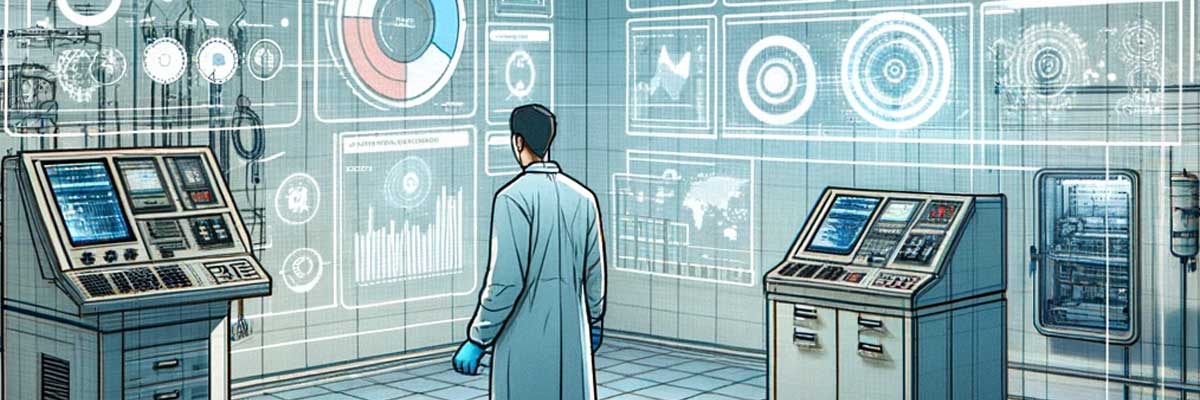
Dos&Donts is committed to continuous improvement of quality and safety standards in the
pharmaceutical industry: this is pursued also through the constant integration of quality services and
advanced technologies, an essential synergy for maximum operational safety, efficiency and process
optimization.
From this perspective, the new Dos&Donts Sigma Cloud Orchestrator collects, controls, processes,
displays and records information from connected entities, integrating them making easy the set up
of preventive maintenance programs.
Within the complex panorama of pharmaceutical production, strict adherence to regulations is
paramount to guarantee the quality, safety and effectiveness of sterile products. Maintenance
assumes a pivotal role in this context and predictive maintenance implementation stands out as an
advanced and effective strategy to enhance compliance with Annex 1. In this short article, we
examine how predictive maintenance can be a crucial support in ensuring smooth operational
processes and compliance to regulatory mandates.
1. Product Quality Assurance:
To the purpose of Annex 1 compliance, predictive maintenance in pharmaceutical production applies an advanced methodology to safeguard product quality by continuous equipment monitoring. This involves deploying sophisticated sensors, data acquisition systems and advanced analysis algorithms to establish a real-time control system.
- Strategic Sensors: : Sensors are placed at critical points in the production process, such as sterile chambers, mixing tanks, or bottling areas. These sensors can detect key parameters such as temperature, humidity, pressure and other indicators specific to the production of sterile pharmaceuticals.
- MContinuous Monitoring: Data collected by sensors are transmitted real-time to a centralized monitoring system. This system can be based on a cloud platform or local servers and represents the core of predictive control.
- Advanced Analysis Algorithms: Analysis algorithms work in tandem with collected data, using predictive models to anticipate any deviations or potential issues. These algorithms can be based on machine learning techniques, automatic learning or advanced statistical analysis.
- Timely Alert Systems: When algorithms identify anomalies or deviations, timely alert systems are activated. These alerts can be sent to maintenance personnel, allowing them to intervene promptly before the situation affects product quality.
2. Reduction of Failure Risk:
Using cutting-edge artificial intelligence (AI) and machine learning methodologies, predictive maintenance proactively detects early indications of failures in equipment employed in pharmaceutical production. These algorithms analyze complex and dynamic data from diverse sources, providing a comprehensive insight into the operational conditions of the equipment. Now, let's explore how these algorithms operate to mitigate the risk of failures.
- Multiple Data Analysis: Predictive maintenance algorithms do not rely on single data sources but integrate information from various sources. These may include vibration, temperature, pressure and flow data together with other relevant variables. Integrating multiple data provides a more complete and accurate view of equipment status. For example, an algorithm can simultaneously analyze pump vibration data and pumped fluid temperature. If vibration increases in conjunction with a temperature rise, the algorithm may identify a potential issue related to wear or overheating.
- Machine Learning from Historical Models: Machine learning algorithms learn from equipment historical data. They study behavior patterns and correlations between various variables to identify early warning signals of failures. This continuous learning process allows algorithms to improve their accuracy in predicting failures over time.
- Real-time Monitoring: Predictive maintenance algorithms operate in real-time, allowing constant monitoring of equipment throughout the entire operational cycle. This feature enables the rapid identification of changes in operating conditions, improving response timeliness.
- Creation of Predictive Models: Algorithms develop predictive models based on analyzed data. These models provide an estimate of the probability of failure within a given time period. This approach allows more accurate planning of maintenance activities, minimizing the impact on production.
3. Maintenance Planning:
Algorithms for predictive maintenance planning play a crucial role in guaranteeing operational efficiency and regulatory compliance, Annex 1 included, in pharmaceutical production. These algorithms use operational data and historical information to precisely anticipate the timing of maintenance interventions, optimizing equipment reliability and minimizing disruptions to production processes.
- Historical Data Analysis: Algorithms start the analysis by examining historical data related to equipment performance. This data includes information on previous maintenance interventions, failure times and operating conditions when failures occurred. The goal is to identify trends and degradation patterns that may indicate when a particular component or system will require maintenance.
- Operational Trends and Degradation Models: : Algorithms analyze operational trends over time, identifying variations in equipment performance. Additionally, they can develop degradation models based on how certain components tend to deteriorate over time. These models consider variables such as usage frequency, environmental conditions and equipment specifications.
- Customized Planning: Once algorithms have gained a deep understanding of historical data, trends and degradation patterns, they can create customized maintenance plans for each piece of equipment. These plans take into account the expected lifecycle of each component, indicating when failures or deteriorations requiring maintenance are likely to occur.
- State-based Maintenance: Predictive maintenance planning goes beyond traditional preventive maintenance. Instead of relying on fixed time intervals, maintenance is scheduled basing on equipment actual conditions. Algorithms constantly assess real-time operational status, and when they detect deviation signals from optimal conditions, they activate alerts and schedule maintenance interventions.
- Resource Optimization:: This planning approach allows optimal use of resources, as maintenance activities are performed only when truly necessary. This minimizes unplanned downtime and associated costs, enabling companies to allocate resources more efficiently and focus on priority interventions.
- Integration with Advanced Monitoring Technologies: To implement effective predictive maintenance planning, it is crucial to integrate advanced monitoring technologies. State-ofthe-art sensors, data acquisition devices and telemetry systems provide real-time inputs to fuel analysis algorithms. The choice and strategic placement of these sensors are essential to obtain representative data sensitive to changes in equipment performance.
- Predictive Maintenance Analytics: Predictive maintenance analytics algorithms often use machine learning and advanced statistical analysis techniques. Predictive models continually adapt to new data, refining their ability to identify failure patterns and predict when maintenance will be needed. Predictive maintenance is not a static approach; rather, it is a dynamic system that evolves with the accumulation of new data and information.
- Proactive Interventions: : Predictive maintenance planning allows proactive interventions, addressing issues before significant failures or operational problems occur. For example, if predictive analysis suggests that a bearing is starting to show signs of wear, operators can replace the bearing during a scheduled maintenance window instead of waiting for an unexpected failure.
- Implementation of Integrated Control Systems: For predictive maintenance planning to work optimally, it is essential to implement integrated control systems. These systems enable bidirectional communication between analysis algorithms and the equipment itself. For example, a control system may receive alerts from a predictive algorithm and automatically activate scheduled maintenance procedures.
4. Documentation and Traceability:
Within predictive maintenance context, the essential role of documentation and traceability are crucial in showcasing adherence to regulatory standards, including those outlined in Annex 1. Advanced systems driven by algorithms facilitate meticulous recording of each maintenance intervention, ensuring a comprehensive record of executed activities.
- Automatic Data Logging: Whenever a predictive maintenance intervention is performed, sensors and diagnostic tools automatically record a wide range of data. This data includes operational parameters, environmental conditions, analysis results and any corrective actions applied.
- Integration with Continuous Monitoring Systems:: Data recorded during maintenance interventions are integrated in equipment continuous monitoring systems. This allows a comprehensive view of operational conditions, highlighting any trends or anomalies that may be correlated with the maintenance intervention.
- Secure Storage and Easy Access: Recorded data are stored securely and in an organized way, allowing quick and reliable access in case of regulatory inspections. Predictive maintenance management platforms use secure cloud-based archives or on-premise systems, ensuring data integrity and availability.
- Automatic Generation of Compliance Reports: Algorithms integrated into management platforms can automatically generate detailed reports documenting everystep of predictive maintenance interventions. These reports are structured in compliance with regulatory requirements and can be presented as tangible evidence of compliance during inspections and audits.
5. Resource Optimization:
Maximizing resource efficiency stands as a critical benefit provided by predictive maintenance in the context of pharmaceutical production. This efficiency is attained through a thorough analysis of operational data to identify patterns, trends and anomalies, thereby enabling proactive asset management and efficiency optimization.
- Downtime Reduction: Predictive algorithms actively work to predict when a machine or specific component will require maintenance, allowing timely interventions before critical failures occur. This anticipation drastically reduces unplanned downtime, increases equipment availability and ensures continuous production.
- Extension of Equipment Lifespan: Thanks to predictive analysis, it is possible to optimize equipment usage, extending its lifespan through targeted maintenance interventions. This results in long-term savings and increased efficiency of maintenance investments.
- Customized Planning of Interventions: Predictive maintenance algorithms can adapt to the specific needs of each piece of equipment. Considering historical data, current operating conditions and degradation trends, customized maintenance interventions can be planned, optimizing resource use.
- Reduction of Maintenance Costs: : Predictive analysis allows targeted resource management, reducing costs associated with maintenance. Timely interventions, customized planning, and prevention of critical failures contribute to optimizing maintenance budgets.
Some Examples:
- Suppose we have a pharmaceutical production machine that uses a critical pump for liquid transfer. Algorithms analyze historical data related to pump performance, constantly monitor pressure, flow and other operational parameters. When algorithms detect a decrease in pump capacity or an increase in vibrations, they trigger a maintenance notification and plan the intervention. This process is based on in-depth data analysis, ensuring that maintenance is performed exactly when needed, minimizing the risk of failures and ensuring compliance with regulations such as Annex 1.
- Imagine a pharmaceutical plant with a critical drying system. Predictive maintenance algorithms constantly monitor temperature, humidity and airflow speed. If predictive analysis detects a gradual temperature increase beyond established limits, the system initiates a maintenance alert. This alert automatically activates a scheduled maintenance window during which operators can perform checks, replace critical components and ensure the proper functioning of the system.
- During a maintenance intervention on a filtration system used in pharmaceutical production, sensors record filter pressure before and after the intervention, detected contamination levels and information on particular substances captured by the filter. If an anomaly in filter performance is detected during the intervention, the documentation system can show in real-time the impact of this correction on the overall performance of the filtration system.
- During a regulatory inspection, inspectors may require specific details about maintenance interventions on particular equipment. The management platform provides immediate access to all data, including detailed reports, photographic document and analysis results.
- If a machine experiences peak usage during certain periods of the year, the algorithm can adjust maintenance planning to be more intense during periods of lower production, avoiding significant impacts on the production chain. An algorithm can suggest that the preventive replacement of a critical component is more cost-effective than dealing with the emergency costs associated with a sudden failure, including downtime and lost production.
The seamless integration of predictive maintenance into pharmaceutical production processes plays a pivotal role in ensuring compliance with Annex 1. By continuous monitoring, prevention of failures and strategic planning, companies not only meet regulatory requirements but also enhance the quality and reliability of their production processes.
Featuring a Machine Learning algorithm, the Dos&Donts Sigma Cloud platform exploits fieldcollected data and statistical analysis to generate crucial "predictions" for the preventive maintenance of connected devices, the data generated from which are systematically stored as big data.
Another step toward safety, quality and excellence in pharmaceutical production, in the direction indicated by Annex1.